Do You Really Need a Randomized Controlled Trial?
A Short Guide to Alternative Research Methodologies
February 22, 2024
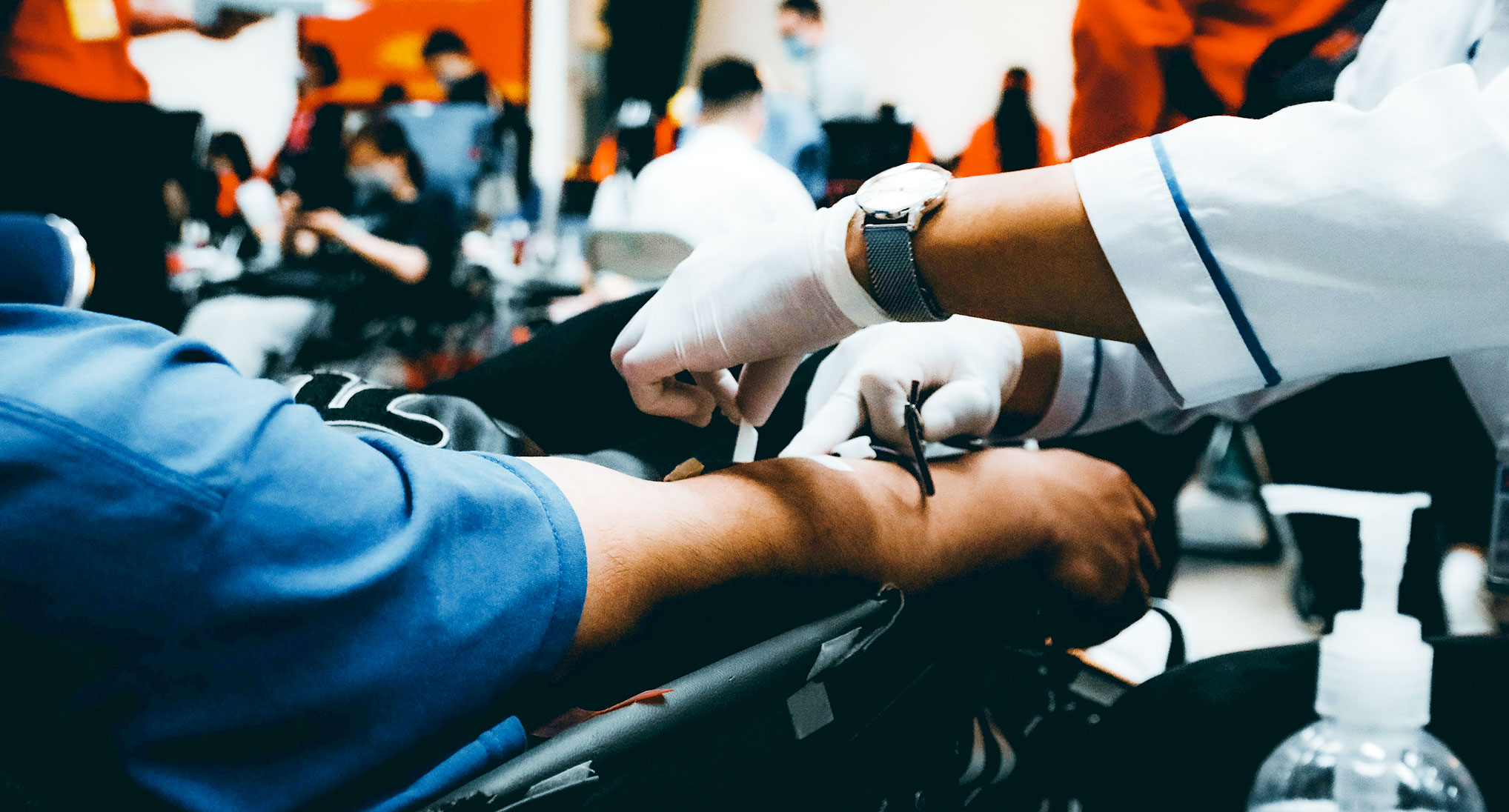
Consider a situation where a healthcare company is trying to assess the cost-effectiveness or the clinical efficacy of a new therapy. How does it choose the most appropriate study design? And how can it ensure that its study is as free as possible of potential biases that may invalidate the results?
The gold standard for research studies of this kind is the randomized controlled trial, in which subjects are randomly assigned to either a treatment group (also known as an exposure group) or to a control group, and the outcomes for the two groups are measured, compared, and analyzed. There is just one problem, and it’s a big one. Not all research questions can be effectively or appropriately addressed in randomized controlled trials. Conducting them also requires a major commitment of resources. Due to the nature of the study or a lack of resources, they may be impractical, inadvisable, or infeasible.
In this issue brief, we look at alternative study designs and methodologies that companies can employ when they cannot or should not conduct a randomized controlled trial. We also examine common biases in research studies and discuss various techniques that can be used to mitigate them.
An Overview of Study Designs
Why has the randomized controlled trial become the gold standard in clinical research?
There are three reasons. The first is that it is easier to mitigate potential biases in randomized controlled trials. Through the randomization process, biases (whether in the selection of study subjects, investigators’ prior assumptions, or the research environment) tend to affect the exposure group and the control group in similar ways and can thus be controlled and minimized. Second, because biases are better controlled, randomized controlled trials provide the surest means of achieving both internal validity (the relationships found in the study reflect true relationships in the target population) and external validity (the results of the study can be applied to similar populations in different settings).1 Finally, because randomized controlled trials allow investigators to actively manipulate exposures (a term that can refer to the characteristics of study subjects as well as the interventions to which they are exposed) and analyze the associated outcomes, they are usually considered the most reliable way for investigators to establish causal relationships.
In randomized controlled trials, blinding is one of the techniques used to minimize biases, and is recommended whenever possible. In a single-blinded design, either the investigators or the subjects do not know who has been assigned to the exposure group and who has been assigned to the control group. In a double-blinded design, neither the investigators nor the subjects know who has been assigned to which group. In a triple-blinded design, even the data analysts do not know how the assignments were made. The views of investigators and study subjects about the research hypothesis being tested may affect the study results. Blinding minimizes this risk.
While randomized controlled trials clearly have many advantages, they are not always possible or desirable. Fortunately, there are alternative study designs to which companies can turn that are also capable of addressing potential biases and providing valid answers to their research questions.
According to the Centre for Evidence-Based Medicine, analytical research studies can be classified into two broad categories: experimental and observational.2 A study is experimental if investigators actively assign subjects to exposure groups and manipulate exposures. It is observational if they simply analyze exposures and outcomes in preexisting or naturally forming groups.
Types of Study Designs
There are two types of experimental studies, the first being the randomized controlled trial. If the assignment of subjects to an exposure group and a control group is not random because randomization is not logistically feasible or ethically acceptable, then we have the second type: a non-randomized controlled trial. For an example of when a non-randomized controlled trial may be necessary, consider a situation where a company wants to assess the effectiveness of a new software platform in managing healthcare costs. The software platform would normally be tested by implementing it on a pilot site, and a random assignment of patients to that site may not be logistically feasible. If assigning certain types of patients to the site could prove disruptive to their care, then randomization would also be ethically unacceptable.
There are three types of observational studies: the cohort study, the case-control study, and the cross-sectional study. In a cohort study, investigators follow study subjects with different exposures and then measure and compare the outcomes of interest. In a case-control study, they start with a group of study subjects exhibiting certain outcomes (the “cases”) and a group without those outcomes (the “controls”), then analyze the exposures in each group and relate them to the differences in outcomes. Both types of study are longitudinal, with cohort studies typically prospective, meaning that the outcomes are measured after the study commences, and case-control studies typically retrospective, meaning that the outcomes have occurred before the study commences. A comparator group is frequently used with cohort studies and case-control studies.
In cross-sectional studies, exposures and outcomes are measured at the same time. These studies are typically used to assess the prevalence of a condition or give a snapshot of relationships between exposures and outcomes. Surveys are a popular form of cross-sectional study.
To see how observational studies might be used in practice, consider the example of a company trying to assess the cost-effectiveness of a new therapy. Investigators might set up a cohort study in which they follow a group of patients receiving the new therapy and a group not receiving it, then compare healthcare costs for the two groups over a 12-month period. While this study is similar to a non-randomized controlled trial, there is a key difference. In a non-randomized controlled trial, the investigators would assign patients to an exposure group and a control group, whereas in the cohort study the groups arise naturally. If the company wants to understand the specific factors that contribute to the cost-effectiveness of the new therapy, it could then select a group of patients for whom the new therapy is cost-effective and a group for whom it is not and analyze the characteristics of the two groups. This would be a case-control study.
Observational studies, if properly designed to address potential biases, can provide robust answers to many healthcare research questions. A well-known example is the Framingham Heart Study.1 Launched in 1948, it is a long-term, large-population cohort study that has greatly contributed to our understanding of the relationship of risk factors to cardiovascular disease.
In the following two sections, we discuss the key questions that companies should consider in selecting an appropriate study design, as well as the different types of biases that can distort study results and how to mitigate them. The following table summarizes the relative strengths and weaknesses of the different types of studies discussed.
Strengths and Weaknesses of Study Designs
Choosing an Appropriate Study Design
In designing any analytical research study, the essential first step is to develop a clear statement of the study’s scope and objectives, including the main research questions that the study is intended to address, the expected relationship between exposures and outcomes, and the possible limitations of its conclusions. Once this statement has been developed, the next step is to choose an appropriate study design. In some cases, an experimental study will be essential. But in others, an observational study may be sufficient—or even preferable. As they review the alternatives, companies should consider the following questions:
- Will the study design raise ethical concerns? It is generally considered unethical to expose study subjects in a clinical trial to known harms or to deprive them of beneficial treatments. For example, in studying the relationship between air pollution and lung disease it would be unethical to intentionally expose study subjects to air pollution. In such cases, an observational study would be more appropriate than an experimental study.While the answers to some ethical questions are straightforward, others are more complex. Suppose that during the course of a randomized controlled trial it becomes clear that certain patients in the exposure group are not responding favorably to a new therapy. Should the trial be terminated for these patients so that they can be treated with other therapies that are known to be effective? Or what if it becomes clear during the course of the trial that the treatment being studied is life-saving? The question here is whether it would be ethical to continue withholding it from the control group.
Such ethical questions often arise in experimental studies, and how they will be answered should be considered at the study design stage. If it seems likely that ethical questions will arise that cannot be satisfactorily addressed, an observational study may be the better choice.
- Can exposures be effectively controlled? The effectiveness of clinical trials hinges on the ability of investigators to manipulate and vary exposures. When exposures cannot be effectively controlled, an observational study may be the best option. Their inability to control exposures is why investigators commonly use cohort studies to examine such questions as the relationship between genes and disease or sexual habits and disease.
- Does the study involve multiple exposures or more than one outcome of interest? Randomized controlled trials work best when the goal is to test a specific hypothesis and measure a primary outcome. While it may be possible to measure secondary outcomes in a randomized controlled trial, the conclusions will not have the same statistical significance as those for primary outcomes. If the goal of the research is exploratory—that is, to study multiple exposures and outcomes and to develop hypotheses for further research—an observational study might be the better design choice.For example, a company with a new therapy may want to study which patient characteristics make the therapy most cost-effective. To do so, it could begin with a cohort study of a group of patients who have received the new therapy and assess how various “exposures” affect outcomes of interest, such as the utilization of medical resources following treatment. The exposures might include age, gender, BMI, disease status, smoking status, and other lifestyle factors. If the company then wants to focus on the relationship between a specific exposure and the effectiveness of the new therapy, it could follow up the cohort study with a randomized controlled trial.
- Is the studied condition rare or slow-developing? A case-control study may be the only practical option when studying rare conditions or conditions that take a long time to develop. Because case-control studies begin with known cases, they avoid the time and expense of identifying rare conditions in large populations or following the experience of study groups over extended periods of time that would be necessary in other types of studies.
- Does your company have the resources to undertake the study? Cross-sectional and case-control studies are generally less expensive and less time-consuming than cohort studies. Cohort studies, in turn, usually require fewer resources and less time to set up and run than randomized or non-randomized controlled trials. Depending on the research question, observational studies alone may be sufficient to give satisfactory answers. And even when they are not, they can play an important role in assessing whether more resource-intensive experimental studies are necessary.
Dealing with Potential Biases
In choosing an appropriate study design, companies will also need to consider whether the design will allow them to mitigate potential biases. Biases can come from expected and unexpected sources. Here we discuss the most frequently encountered biases, using the example of a company that wants to assess the cost-effectiveness of a new therapy by measuring the 12-month healthcare cost for a group of study subjects before and after the therapy is introduced.
- Confounding variables. Confounding variables are variables that are not studied exposures but nonetheless affect the outcomes being measured. When they are present, the results of the study may not accurately reflect actual relationships between studied exposures and outcomes. In our example, if the healthcare cost for the 12-month period prior to the introduction of the new therapy has been skewed upward by an unusually severe flu season, a reduction in the 12-month healthcare cost following its introduction may not be indictive of the cost-effectiveness of the new therapy but simply reflect the healthcare cost of a normal year. In this case, the severity of the flu season is the confounding variable.Not all confounding variables can be controlled, measured, or known. But if a control group is included in the study design and the confounding variables are distributed similarly between the exposure group and the control group, then the problem can be minimized. While this is easier to do in experimental studies, it is also possible in observational studies. In a cohort study, for instance, the effect of confounding variables can be reduced by matching the characteristics of the exposure group with those of a comparator group and by studying both groups under similar external environments and over similar time periods.
- Regression toward the mean. The regression toward the mean phenomenon was first described in 1886 by Frances Galton, who found that parents whose height was above average tended to have children who on average were not as tall as they were. Average height thus regressed toward the mean over time. This phenomenon can arise in research studies due to random variations inherent in the observations. Consider our example of 12-month healthcare cost before and after the introduction of a new therapy. If the 12-month cost prior to the introduction of the therapy was a higher-than-average observation, a reduction in the 12-month cost after its introduction might be due to natural regression toward the mean rather than the effectiveness of the new therapy.The risk that regression toward the mean may skew results is typically mitigated through randomization and a sufficiently large sample size. To mitigate the risk without a randomized controlled trial, investigators could perform multiple measurements of healthcare cost before and after the introduction of the new therapy, increase the time periods over which healthcare cost is measured, or increase the sample size in the study.
- Placebo effects. It is well known that the condition of patients sometimes improves because of their perception of the effectiveness of a healthcare intervention, rather than because of the intervention itself. This phenomenon is known as the placebo effect. In our example, let’s assume that the 12-month healthcare cost declines after the introduction of the new therapy. The challenge for investigators would be to determine whether the decline is due to the effectiveness of the therapy or to study subjects’ perception of its effectiveness, which might lead to behavioral changes that similarly reduce costs. Placebo effects are typically mitigated by blinding the study subjects. When blinding is not possible, investigators will need to carefully review the study design for potential placebo effects and determine whether the risk that they will affect the results is acceptable.
- Selection bias. Selection bias arises when the method of recruiting subjects for a research study results in an exposure group whose characteristics are different from those of the target population of which the exposure group is meant to be representative. When this is the case, the relationships observed among study subjects may not be applicable to the target population as a whole. In our example, the company evaluating the cost-effectiveness of a new therapy may have carried out its study in a hospital with a high percentage of Medicaid coverage. If so, the results of the study may not be applicable to a target population with a lower percentage of Medicaid coverage. Similarly, if an exposure group and a control group are recruited from different hospitals with different percentages of Medicaid coverage, a comparison of the two groups’ 12-month healthcare cost may not be valid. To reduce selection bias, investigators should compare and match relevant characteristics of the exposure group and the target population.
- Response bias. Response bias can occur when data are collected from voluntary respondents whose characteristics may not reflect those of the target population or when data are collected through subjective assessments or self-assessments that may not be accurate. In our example, if collecting 12-month healthcare cost data after the study has been completed requires the study subjects to sign a new consent form, there may be response bias if those subjects with better outcomes are more willing to sign the new form than those with worse outcomes. To reduce response bias, investigators should review data collection processes and analyze factors that may affect subjects’ response rates.
- Survivorship bias. It is often the case that not all subjects finish a study. If the withdrawing subjects tend to have more or less favorable outcomes than average, then there may be survivorship bias. In our example, if the withdrawing subjects tend to be those with relatively higher healthcare costs, then the reduction in the 12-month healthcare cost will be biased higher, causing the observed impact of the new therapy to appear more favorable than the true impact. To remove survivorship bias, investigators need to monitor withdrawing subjects and potentially conduct additional statistical analysis.
Randomized controlled trials are often better at mitigating these biases than other types of studies, but they are by no means immune to them. A cohort study that is well-designed and well-run may yield more valuable information than a poorly executed randomized controlled trial. All types of research studies, moreover, can enhance internal validity by employing various standard techniques, including using a control group, matching and monitoring key characteristics of subject groups, ensuring a sufficiently large sample, and using appropriate methods of recruitment, data collection, and analysis, as well as randomization and blinding whenever possible.
Conclusion
Randomized controlled trials may be the gold standard in research studies, but they are not the only useful study design and in some cases may be impractical, inadvisable, or infeasible. Provided that they adequately address potential biases, the alternative study designs discussed in this issue brief can achieve a level of internal validity suitable for decision-making while providing robust evidence-based answers to many healthcare research questions.