Algorithmic Accountability: What Is It and Why Does It Matter?
February 23, 2023
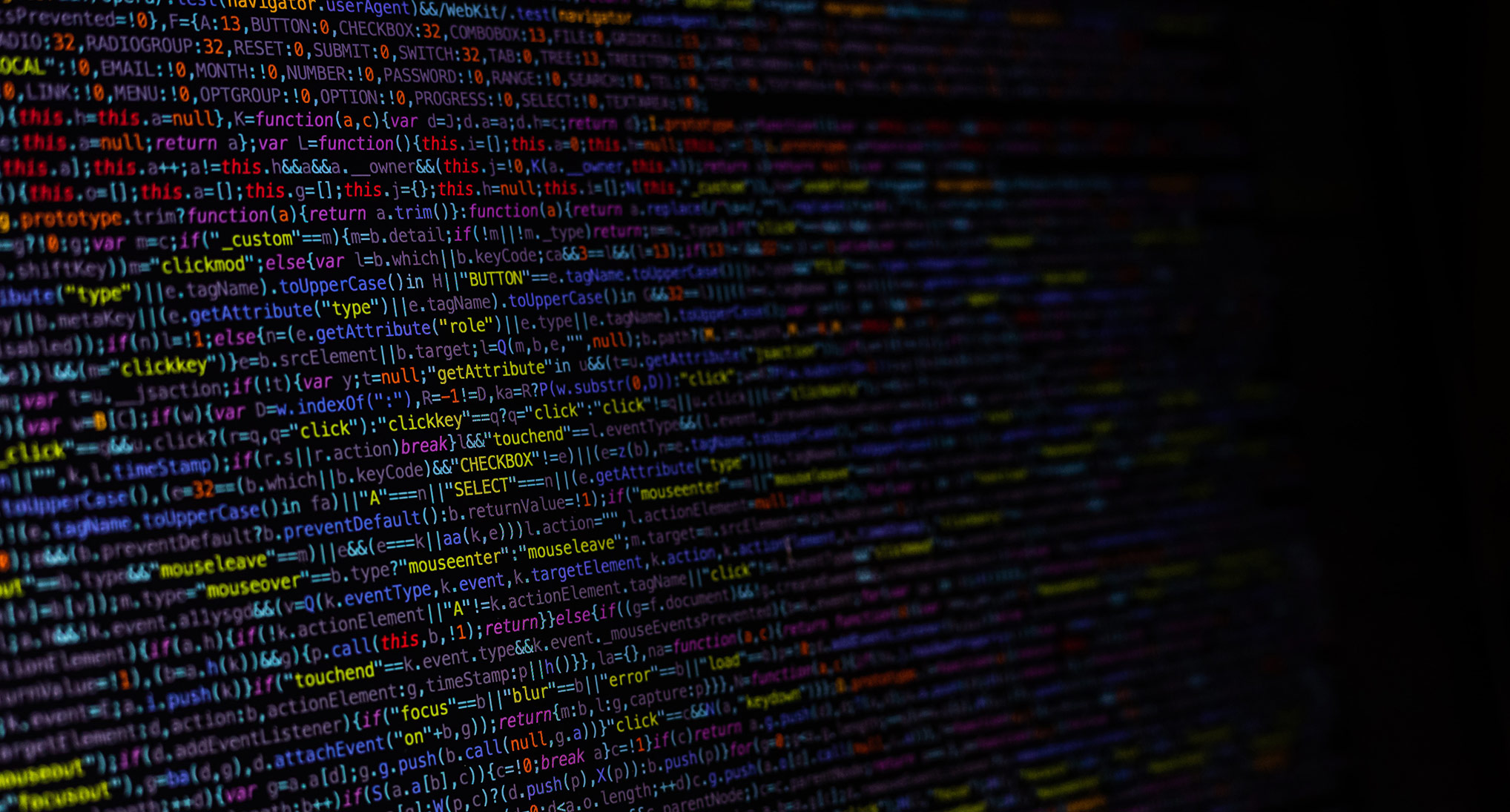
Key Learnings
- In this primer on algorithmic accountability, we discuss how accelerating usage of algorithms has brought heightened scrutiny that impacts businesses employing algorithms and AI systems.
- Businesses that are not proactive with their own algorithm vigilance, governance and guidelines are only delaying the inevitable and neglecting potentially devastating risk and reputational exposure.
- The integrity of underlying algorithms is coming under ever-tightening focus through algorithm accountability standards and regulations.
- Examining these guidance documents across various countries and industries, seven common facets of accountability emerge: reliability, safety, concern for fairness and bias, security and resilience, transparency, explainability and interpretability, and privacy/data governance.
- We list the main algorithmic accountability policies that are being implemented around the world.
ChatGPT is here and, boy, has it captured our attention. The dynamic v1.0 AI-powered question-response tool has demonstrated how far we have come in replacing our reliance on humans with algorithm-driven engines.
It’s a compelling example of AI possibility, but by no means is this an algorithmic genesis. Indeed, algorithms have been powering aspects of the business world for quite some time, from insurance to credit cards.
Algorithm usage is everywhere, but until ChatGPT arrived, they had simply been running more quietly in the background. So quietly, in fact, that corporate leaders may not be fully aware of how widely their own business relies on algorithms, nor have they needed to pay attention.
But this is all about to change.
AI is Proliferating, and So is the Scrutiny
In 2016, the Arkansas Department of Human Services changed the way it determined the hours of care needed for low-income patients with disabilities—shifting from a nurse assessment of each beneficiary to an algorithm. Consequently, many recipients suffered insufficient care, sometimes “leaving them with bedsores or lying in their own waste,” according to a lawsuit brought and won by the ACLU.
One of the most novel aspects of this case was the source of fault: it wasn’t a person, it was an algorithm.
Algorithms guide decisions about who gets what—what retail ads, TV show recommendations, health resources, mortgages or legal privileges. Beyond greater speed and efficiency, the appeal of using mathematic formulas is the notion that they are neutral arbiters of truth.
After all, it’s math.
But while algorithms take objective points of reference and provide a standard outcome, input limitations can cause outputs to be fallible.
So it’s no surprise that, as AI accelerates its influence across governments and industries alike, the integrity of underlying algorithms is coming under ever-tightening focus. Big Tech has been the first to respond—such as Google’s AI principles, Meta’s five pillars of responsible AI and Microsoft’s responsible AI practices —but most industries will eventually follow suit.
Businesses Should Prepare Now
What does this mean for your organization? For business leaders, the time is here to fully uncover where the algorithms lie – across sales, marketing, operations, human resources and finance, both among internal systems as well as those of external partners and vendors. A responsible data analytics partner can help leaders complete this inventory, and develop the standards and controls for accountable data, algorithms and AI systems.
Businesses that aren’t proactive with their own algorithm vigilance, governance and guidelines now are not only delaying the inevitable but neglecting potentially devastating risk and reputational exposure. As we detail below, regulatory algorithmic accountability standards have already emerged in the U.S., Singapore, the E.U. and Canada, among others, and they’re just getting started.
What is Algorithmic Accountability?
Algorithmic accountability is the idea that those designing, procuring, and using flawed algorithms should be held responsible for their actions and the impacts of those algorithms. This can include being accountable for the accuracy and fairness of the algorithm, as well as for any negative consequences that may result from its use.
7 Facets of Algorithmic Accountability
Developing standards for algorithmic accountability has taken different approaches and flavors, but examined together, they boil down to these seven facets:
1. Robustness and reliability
Robustness of data and research underlying the algorithm, accurate results, and the reliability of algorithm outputs are inextricably linked. Robustness, accuracy, and reliability are interdependent factors that contribute to the validity and trustworthiness of AI systems.
We’ve found that most flaws in designing or using algorithms can generally be traced back to two broad root causes:
- Algorithms aiming at the wrong target. For instance, we previously wrote about how payers and providers use area healthcare utilization data for establishing provider networks, calculating risk adjustment scores, and targeting preventative care services, but this data does not necessarily reflect a community’s underlying health risks and needs, particularly among disadvantaged populations.
- Lack of diverse representation in clinical studies or training groups. For example, pulse oximeter devices measure light absorption through the skin and pass the resulting data through an algorithm to approximate a patient’s true blood oxygen levels. Though the algorithm was focused on the right problem, studies have shown that it performed poorly in black patients, likely because it was trained on primarily white patients. This violates the principle that patients with the same score should have the same true need or outcome, irrespective of race.
Algorithmic accuracy cannot be achieved without robust usage of outside data. When an AI system’s scope of information and inputs is too limited, it devolves into a case of “garbage in, garbage out.”
2. Safety
It’s no exaggeration to say that in some cases, algorithms can be arbiters of life and death. AI systems can inform life-altering decisions based on real-time data from sensors, remote inputs, and existing data with minimal or no human intervention. For instance, healthcare is increasingly using AI for cancer detection techniques and the management of congestive heart failure.
If a decision-making algorithm causes physical or psychological harm or endangers human life, health, property, or the environment, its use must be immediately suspended or discontinued until its defects are repaired or a more reliable system is implemented.
3. Concern for fairness and bias
In 2018, Amazon built an AI recruiting system to read resumes and select the strongest candidates based on past resume data. However, the tool was determined to be discriminatory against women because the 10 years of resumes used to shape the machine’s learning were predominantly submitted by males.
Addressing algorithmic bias and maintaining impartial AI systems requires attentiveness to building equality and equity as well as concern about the detrimental outcomes of an inherently discriminatory system.
Screening for bias and discrimination and maintaining algorithmic oversight requires vigilance. Designating a steward who works in close collaboration with a diverse committee of internal and external stakeholders is common practice for addressing this.
4. Security and resilience
In this context, security and resilience refer to the ability of AI systems to:
- withstand adversarial attacks or, more generally, unexpected changes in their environment or use
- maintain their functions and structure in the face of internal and external change
- degrade gracefully when this is necessary
AI systems are becoming more powerful, impactful, and far-reaching than ever before. As we become more reliant on them for life-altering decisions, these systems need to be capable of remaining stable, uncompromised, and trustworthy.
5. Transparency and accountability
Transparency reflects the extent to which information is available to individuals about an AI system with which they are interacting.
Frank Pasquale, a law professor at the University of Maryland, calls the algorithmic decision process a “black box,” meaning that while we may know what data goes into the computer for processing and the outcome it spews out, there are scant third-party auditing systems or regulations for being able to see what happens to the data during processing.
This is a prevalent issue in the criminal justice system, where “affected people are often unable to know what tools the jurisdictions they live in use because of trade secret carveouts in Open Government laws, as well as similar roadblocks in evidentiary and discovery rules,” according to the Electronic Privacy Information Center.
Not only is the public unaware of the inner workings of AI systems; even people operating the systems may be ignorant of the mechanisms of the process…or whether the results are accurate.
Agencies sometimes acquire algorithms without fully understanding how they function or assessing their reliability, and then often fail to test their reliability in a real-world setting.
On a related note, when algorithms fail to protect the safety and well-being of those involved, parties using those algorithms may be held liable for neglecting to assess the accuracy of their AI systems—or worse, continuing to use a flawed system.
6. Explainable and interpretable
Those who build, procure, and use algorithms should be able to give an accurate representation of the mechanisms underlying an algorithm’s operation—i.e., the data it uses and how it produces different outcomes. The National Institute of Standards (NIST) distills AI explainability to four principles:
- Explanation: The system gives evidence or reasoning for all outputs.
- Meaningful: Individual users have access to meaningful explanations of the system.
- Explanation Accuracy: The system explanation matches its process for generating the output.
- Knowledge Limits: The system is only used for what it was intended.
Interpretability, on the other hand, refers to the meaning of AI systems’ output in the context of its designed functional purpose—basically, the course of action a person will take in response to the algorithm’s result.
7. Privacy/data governance
Privacy is generally considered the norms and practices that help to safeguard human autonomy, identity, and dignity. Safeguarded privacy data may be tempting to use for certain machine learning problems, particularly in light of the data robustness factor above, which is why it’s critically important to clearly define privacy data usage limitations.
Like algorithm accountability, regulations protecting personal privacy continue to grow and for users of data, tighten. The California Consumer Privacy Act (CCPA) came into effect in 2020 and, since then, more than 300 cases have been filed by plaintiffs. Similar privacy protection laws have more recently been enacted in Colorado, Connecticut, Virginia and Utah.
Algorithmic Accountability Policies Being Implemented Around the World
As more industries come to rely on algorithm-based decision-making, many government entities and industries have introduced algorithmic accountability and AI policies with the expectation of compliance from those who build, procure, and use algorithms. A list of select policies is below (updated February 2024).
We plan to keep this as a ‘living document’ that is updated periodically as new policies and standards are known. If you know of a relevant policy, guidance document or standard that you would like to be added to the list, please email us at insights@terrygroup.com.
Title | Summary | Country/Year | Status |
---|---|---|---|
Colorado Algorithm and Predictive Model Governance Regulation | Establishes requirements for a life insurance company’s internal governance and risk management framework to ensure the use of external consumer data and information sources, algorithms, and predictive models does not result in discriminatory practices. | U.S., 2023 | Passed |
NIST AI Risk Management Framework | Provides a disciplined and structured process that integrates information security and risk management activities into the system development life cycle. | U.S., 2023 | Published |
The White House’s Blueprint for an AI Bill of Rights | Identifies five principles that should guide the design, use, and deployment of automated systems to protect the American public in the age of artificial intelligence: 1) safe and effective systems 2) algorithmic discrimination protections 3) data privacy 4) notice and explanation, and 5) human alternatives, consideration, and fallback. | U.S., 2022 | Published |
Artificial Intelligence and Data Act | Regulates certain AI systems and ensures measures are adopted to mitigate various risks of harm biased output, and also establishes prohibitions related to the possession or use of illegally obtained personal information for an AI system if its use causes serious harm to individuals. | Canada, 2022 | Proposed |
Algorithmic Accountability Act of 2022 | Requires companies to assess the impacts of the automated systems they use and sell; creates new transparency about when and how automated systems are used; and empowers consumers to make informed choices about the automation of critical decisions. | U.S., 2022 | Proposed |
New York City Automated Employment Decision Tools | Clarifies the requirements for the use of automated employment decision tools within New York City, the notices to employees and candidates for employment regarding the use of the tool, the bias audit for the tool, and the required published results of the bias audit. | U.S., 2022 | Passed |
GAO Artificial Intelligence: An Accountability Framework for Federal Agencies and Other Entities | Identifies key practices to help ensure accountability and responsible AI use by federal agencies and other entities involved in the design, development, deployment, and continuous monitoring of AI systems. | U.S., 2021 | Published |
Washington, D.C. Stop Discrimination by Algorithms Act | Protects against discrimination by automated decision-making tools and gives Washington D.C. residents transparency about how algorithms are used to determine outcomes in everyday life – including in credit, housing, and employment. | U.S., 2023 | Proposed |
Artificial Intelligence Act | Comprehensive AI law. Assigns applications of AI to three risk categories: 1) applications and systems that create an unacceptable risk are banned 2) high-risk applications are subject to specific legal requirements 3) applications not explicitly banned or listed as high-risk are largely left unregulated. | Europe, 2021, 2022, 2023 | Proposed |
Model AI Governance Framework | Model framework to help organizations deploy AI responsibly. Aims to help organizations promote public understanding and trust in technologies by explaining how AI systems work, building good data accountability practices, and creating open and transparent communication. | Singapore, 2020 | Published |
U.S. Department of Defense’s Ethical Principles for Artificial Intelligence | The Defense Department formally adopted five principles for the ethical development of artificial intelligence capabilities: responsible, equitable, traceable, reliable, and governable. | U.S., 2020 | Adopted |
G7 Leaders Agreement on International Guiding Principles for Advanced AI Systems and Code of Conduct for Developers under the Hiroshima AI Process | Promotes safe, secure, and trustworthy AI worldwide and provides guidance for organizations developing and using the most advanced AI systems. Calls on organizations to identify, evaluate, and mitigate risks across the AI lifecycle; identify and report patterns of misuse after deployment; prioritize security and transparency; and advance the development of problem-solving AI systems that adhere to international technical standards. | Multiple, 2023 | Published |
A Comprehensive Framework for Ethical and Responsible Use of AI Systems | Its primary aim is to grant individuals significant rights and place specific obligations on companies that develop or use AI technology. The bill establishes a new regulatory body to enforce the law and takes a risk-based approach by organizing AI systems into different categories. It also introduces civil liability for providers or operators of AI systems, along with a reporting obligation for significant security incidents. | Brazil, 2023 | Proposed |
Internet Information Service Algorithmic Recommendation Management Provisions | This regulates personalized recommendations in mobile applications and requires that algorithm recommendation services providers uphold certain user rights. It also prohibits algorithmic generation of fake news. | China, 2022 | Passed |
Interim Measures for the Management of Generative Artificial Intelligence Services | Encourages innovation, development, and international exchange, while making AI subject to reasonable supervision. It establishes four systems for the provision of generative AI services: 1) graded and categorized supervision 2) service agreements between providers and users 3) regulation on AI provided from outside China and 4) foreign investment in generative AI services. | China, 2023 | Passed |
Principles of Policy, Regulation and Ethics in AI (draft policy) | States that the development and use of AI should respect “the rule of law, fundamental rights and public interests and, in particular, [maintain] human dignity and privacy.” Furthermore, “reasonable measures must be taken in accordance with accepted professional concepts” to ensure AI products are safe to use. | Israel, 2022 | Published |
Proposed Advisory Guidelines on Use of Personal Data in AI Recommendation and Decision Systems | The goal is to clarify how Singapore’s Personal Data Protection Act applies to the collection and use of personal data by organizations to develop and deploy machine learning models or AI systems used to make decisions autonomously or to assist a human decision-maker. | Singapore, 2023 | Published |
UAE National Strategy for Artificial Intelligence | An Artificial Intelligence and Blockchain Council will “review national approaches to issues such as data management, ethics and cybersecurity,” and observe and integrate global best practices on AI. | United Arab Emirates, 2018 | Published |
European Union’s Artificial Intelligence Act | Places restrictions on high-risk AI technologies including biometric systems and facial recognition technology. It also includes new transparency requirements for AI, including identifying when an image is AI-generated. | Europe, 2023 | Passed |
ISO/IEC 42001 AI Management System Standard (AIMS) | Provides guidelines for organizations to evaluate and leverage AI responsibly. The guidance includes protocols for “establishing, implementing, maintaining and continually improving an AI (artificial intelligence) management system.” | Worldwide, 2023 | Published |